AI gone wild: why evil data is scarier than evil algorithms
Paul Goodenough
August 02, 2018
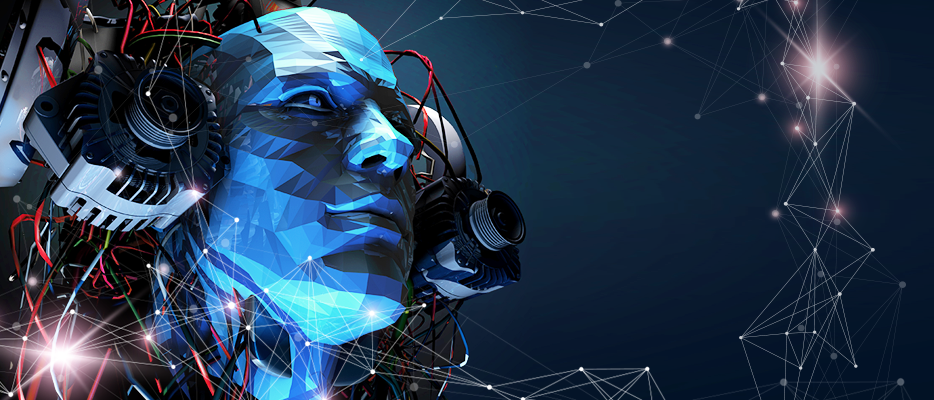
As artificial intelligence (AI) is applied to new sectors of the economy, more and more people are concerned that AI constitutes a real danger: not only to their jobs but to mankind as a whole. Let's take a closer look to see if AI is indeed as scary as some people would have us believe.
Unlocking the origin of fear
Is Artificial intelligence all that scary? Nick Bostrom in his book "Superintelligence: Paths, Dangers, Strategies" professes the arrival of AI that exceeds humans in its capabilities and graphically depicts how, when and why it will destroy us. From 1920, when Karel Čapek first introduced the word "robot" in his play R.U.R., to Westworld in 2018, robots have always rebelled, destroyed or attacked throughout popular culture. In 1970 Masahiro Mori introduced a term "Uncanny Valley" in an attempt to conceptualize our fear of humanoid objects. Whenever something appears almost, but not exactly, like real human beings, it is perceived as uncanny and even repulsive. Are people scared of AI because it exhibits and sometimes exceeds our known level of intelligence? Well, not exactly. Yes, AI can play chess better than you, but so can Magnus Carlsen. Does this make you afraid of the Swedish grandmaster? Probably, not.
Good versus ‘evil’ datasets
But it seems the problem lies elsewhere. People are afraid of the things they do not understand. We were afraid of trains at the end of the 19th century; we were afraid of cars at the beginning of the 20th century; we were (and some of us still are) afraid of airplanes, cell phones, micro-ovens, you name it. Fear is the backside of curiosity. And the only way to make AI less scary is to educate in a clear and simple manner. One of the recent projects about "scary" AI that I loved is Norman (http://norman-ai.mit.edu). Norman is the world's first psychopath AI created by MIT Media Lab and Scalable Cooperation. In essence, Norman is an image captioning artificial neural network that was trained on the "wrong" data, found on Reddit to illustrate how data, that we train algorithm on, affects its performance. The researchers showed Norman inkblots from a Rorschach test and compared the captions generated by Norman with a "normal" image-capturing algorithm. The differences are shocking: where standard AI "sees" a black and white photo of a small bird Norman caption says "man gets pulled into dough machine". The lesson here is not new, but is important: biases that intelligence produces are often not rooted in intelligence per se, yet are the consequence of the biased data it was trained on. As simple as that.
It is interesting that this uncomplicated conclusion is also correct in a broader perspective. In any industrial task where you use AI, your algorithm is only as good as the data you train it on. "Evil" data in — "evil" results out. This is why we need to analyze and understand the data we train our algorithms on. We need to detect socially and professionally dangerous biases before we come up with production-ready solutions. The good news is that there are tools that can help us. You guessed right: these are AI and data-analytics. A big part of content intelligence that ABBYY provides is centered around the understanding of unstructured data. This understanding, among other things, helps us curate datasets we use for further training. After all, until general AI is out of our reach, training data is by far the scariest part of AI.