How Machine Learning Masters Unstructured Information
Dr. Marlene Wolfgruber
May 04, 2018
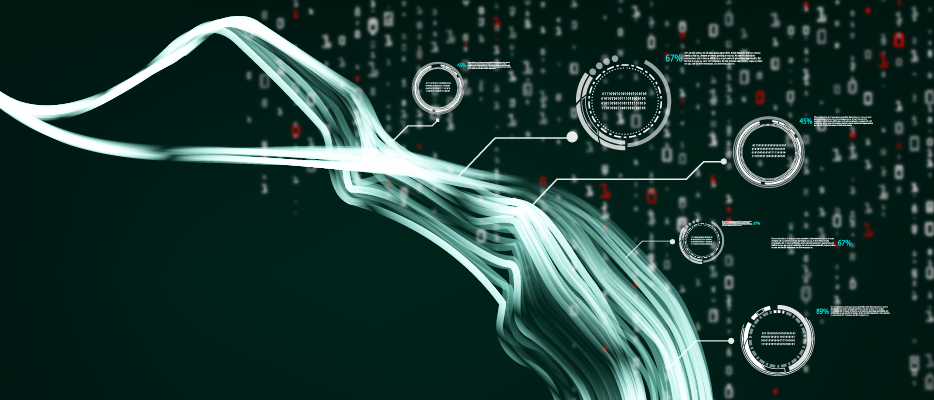
Ever wondered how machines learn to teach themselves to recognize objects, text, spoken words and more? Everywhere, everyone feels overwhelmed by the torrent of information. Yet the vast majority of data people have access to is unstructured, i.e. written in natural language. The main tool for providing companies with reliable access to relevant information is through automatic text classification for organizing and prioritizing unstructured data. Thanks to linguistic and semantic technologies as well as machine learning, the information hidden in big content is now accessible to decision makers and knowledge specialists in companies.
The basic principle of machine learning is the automatic identification and use of the most relevant characteristics from a set of training documents. The system automatically recognizes the common characteristics that make up the documents in a category and uses these characteristics to create the classification model. Various algorithms are automatically tested, evaluated with regard to their performance on the training set and the best model is selected.
Steps for creation and tuning of classification models
1. Configuration and training
The first is to define the categories to which documents are to be assigned. Then from the document stock, select representative documents, which are prototypical for the individual categories. These are required for creating the classification model and checking it. Large quantities of training documents are not required. With the introduction of intelligent classification technologies, a minimum technical number of ten documents for a class, with a minimum of 100 documents will suffice for the generation of reliable statistics. The creation and administration of classification projects and models as well as the upload of training and control documents is carried out either via a graphical user interface or a programming interface.
2. Quality evaluation
Once the training phase is complete, check to ensure that the training process was successful. For this purpose, a control set of documents is loaded into the classification system, classified with the model developed in the training and analyzed with regard to performance. Key figures such as F-measurement and rate of false-positive/false-negative results can be used to assess performance. At this stage, unknown or incorrectly classified documents are reassigned, categories are redefined if necessary or additional documents are added to the training set to improve the results.
3. Implementation
If the classification results of the control set meet expectations and the required quality is achieved, the model can be released for production. Now, unknown texts and documents can be transmitted for classification. The result of classification is metadata that contains information such as the name of the classification model, categories with the corresponding probabilities, confidentiality labels, lists of characteristics/words, plain text, or error messages. JSON or RDF/XML are used as file format. Thus, a metadata collection is created from normal text, which allows much more targeted access to the facts and concepts contained in the text.
Integration of classification in the IT environment
Ideally, an intelligent classification technology is not domain-specific and does not require a hard-coded classification workflow, but rather functions as an independent service in the IT environment of a company. Smart classification modules can be easily integrated into existing IT systems via simple interfaces, such as a REST API, making them intelligent components of archives, content management systems, enterprise search systems, workflows, knowledge bases, email management systems and other software for processing business information.
Application scenarios and added values
Delivering relevant information to the right people at the right time is the key to success in a rapidly changing business world. On the one hand, intelligent classification enables companies to precisely catalog large volumes of data and thus make efficient use of the content in existing systems. On the other hand, incoming documents are classified quickly and reliably, thus making them more usable for further processing and deriving business values from the constantly incoming data stream. Classified information is ready for search and access, automatic routing within the organization and for data extraction.
Classification makes unstructured content accessible, and business-critical information can be localized quickly and efficiently. The transparency created in this way helps to minimize business risks, to fulfill legal requirements for data protection and compliance and to optimize processes. The more transparent and searchable the information, the faster decisions can be made, which contributes to general business agility and customer satisfaction.
This is an abridged version of Dr. Marlene Wolfgruber's article published in the online German magazine Bigdata-Insider. Please click here to read the full version (in German).