Training for Intelligent Performance
June 28, 2018
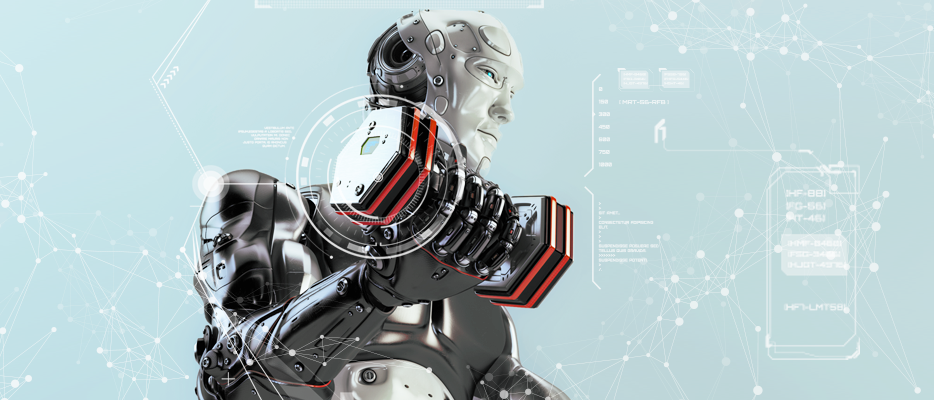
As artificial intelligence (AI) weaves itself into every aspect of production and services, it is time to consider how business could leverage this technology to analyze their massive datasets and drive better business outcomes. We spoke with Dr. Ivan P. Yamshchikov, AI Evangelist at ABBYY about the opportunities and challenges of training AI systems and how they are impacting business outcomes.
Q. Why is it important to train AI systems?
IY. AI systems are assuming increasing importance in all spheres of life. Just to give some examples, AI algorithms assist steel manufacturing, because it makes the production cheaper and safer for environment. AI-based voice recognition is developed so that drivers are not glued to the screens while driving. And, AI is used in the search-engines since you simply cannot effectively handle the massive amount of information on the web in any other way. ABBYY specifically develops AI-solutions to optimize business processes. Our AI helps to reduce bureaucracy, saves time that people spend working with documents and, at the end of the day, makes people more productive and happier. I can tell you that, most of the time when an office-worker "hates his job" it is because the task he performs is repetitive, and involves documents. We use AI to minimize that.
Q. Is knowledge of programming/code writing required in order to train a machine learning system?
IY. It depends on how you define "train". Indeed, a knowledge of mathematics is needed to design a machine learning system and you need to know how to code in order to implement the model correctly, but the whole beauty of machine learning is that as long as the system is up and running, it could learn by itself. Every user can train an AI algorithm if it is properly designed. If the system makes a mistake and you correct it through some form of a feedback, you are actually training the AI.
Q. What type of data are useful for training AI?
IY. There are two big families of algorithms: supervised, that use labelling, and unsupervised, that don't. For example, clustering is a typical example of an unsupervised family of algorithms. Lets say you have several types of documents with different visual layouts, such as invoices or bills. With machine vision technologies, ABBYY FlexiCapture can classify and sort these different documents automatically. Since visual layout is varies according to different types of documents, you can use an unsupervised method and you do not need human labelling. However, if we talk about automated fraud detection or analysis of a contract for compliance, you have to have a dataset of hand-marked examples to train your AI on it.
Q. Are some datasets easier to tag than others?
IY. There are lots of factors that play a role. Generally, the higher the level of human expertise needed to tag certain dataset, the harder and more costly it is. If you have 1 Gb of cats and dogs photos and you want to tag manually, it should be relatively easy. If you have 1 Gb of Japanese manga and you want to tag all the pictures that have adjectives in the lines of the characters, that is way harder to pull off.
Q. Is data classification (as in FlexiCapture) the same thing as "data-tagging" or "data-labelling"?
IY. In a sense, it is. However, we usually talk about labelling or tagging if it is manual and use words clustering or classification when this is performed automatically.
Q. Does one need huge amount of datasets to train Machine Learning systems?
IY. You have to have data to train a model from scratch, but it does not necessarily mean that you need a lot of data to use certain AI-powered product. For example, we have advanced NLP algorithms in ABBYY that could be used for extensive document analysis. To develop these algorithm, we had to work with huge corpora of texts in every language, so that now business can have access to this NLP system and apply it to their datasets. Each of our clients would have to use years of research and developments and tons of data to get the same NLP technology on their own. The good news is that they can use ours for a fraction of that cost.
Q. Which ABBYY technologies rely heavily on ML technology?
IY. Every product, that we currently have, uses machine learning in some form, but that does not mean that you need a lot of data to start using such products. For example, FlexiCapture uses convolutional neural networks for document preprocessing and classification, but just several examples of the documents are usually enough to have the pipeline up and running. Our NLP technologies use a spectrum of machine learning methods and combine them with advanced ontologies for every language, but we can carry out a lot of tasks connected with natural language processing for you without extensive datasets provided to us. So, if you think of any document-related bottlenecks in your current business processes, ABBYY probably has a product for you to make your business faster and simpler, and your employees more effective and engaged.